We recently published an article that presented the benefits of conducting environmental monitoring with remote sensing, offering examples of its application and the main technological trends related to the theme. This article explores applications of remote sensing for monitoring vegetation, how the information sought is generated, what types of vegetation can be monitored remotely and some examples of application in real situations.
Monitoring of vegetation by remote sensing
Monitoring of vegetation is one of the most common types of monitoring to be conducted by remote sensing and can be applied to various situations and needs. Examples of applications that benefit from this type of technology include:
- Monitoring of suppression of vegetation in areas of direct and indirect impact of construction projects;
- Accompanying changes in reforestation projects;
- Monitoring of vegetation to improve management (for ex. close to electrical grids, railroads, green areas);
- Monitoring of environmental compliance in agricultural production chains;
- Inspection of use of agricultural credit on rural properties;
- Monitoring of forest-based production; and
- Monitoring of projects to reduce carbon emissions caused by deforestation and forest degradation (REDD+).
The monitoring of vegetation by remote sensing is conducted mainly with images collected by multi-spectral optical sensors aboard satellites (such as OLI/Landsat-8, MSI/Sentinel-2, MUX e WPM/CBERS-4A), and can also be conducted with images collected by unmanned aerial vehicles (like Horus) and nanosatellites (like Planet) and also with images collected by radar (such as C-SAR/Sentinel-1), that can collect images regardless of weather conditions and cloud cover.
The diversity of sensors and types of platforms for acquisition of images currently available allows observing vegetation on a wide variety of spatial and temporal scales. It is possible to detect and monitor trees individually or examine dense and extensive forests, even in regions that are frequently covered by clouds (such as the Amazon basin):
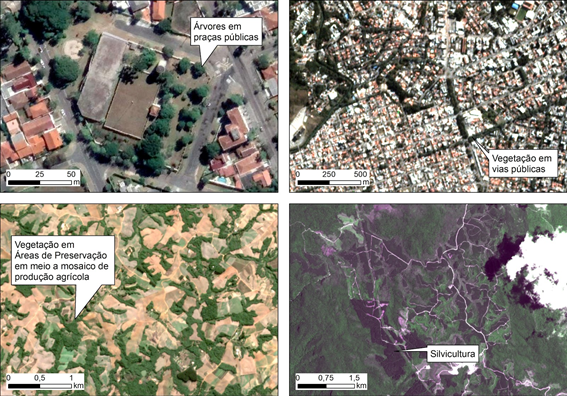
How is vegetation monitored with images and what information can be obtained from these images?
Remote detection of vegetation
The remote detection of vegetation allows verifying its occurrence in a given locality, accompanying its changes over time and quantifying its area and scope, by means of digital interpretation and image processing. To simplify, the detection and monitoring of vegetation based on images from remote sensing can be realized in 2 ways: (1) manually; or (2) automatically.
Manual detection
Manual detection is conducted by an experienced photo interpreter who visually interprets colored compositions of previously processed images. Traits of interests are vectorized with assistance from a Geographic Information System (GIS). The temporal frequency, scale of mapping and spatial scope of the monitoring can make manual techniques inviable, by requiring a large quantity of human and financial resources.
Automatic detection
Automatic detection of vegetation uses algorithms for classification and analysis, highlighted by machine learning (ML) algorithms. ML algorithms that are widely cited in the literature and used for classification and monitoring of vegetation include supervised learning algorithms, such as Support Vector Machine, Decision Trees, Random Forest and Artificial Neural Networks.
These algorithms are now implemented and available in various open source and commercial software (such as ENVI, InterIMAGE and WEKA) and in packages implemented in R language (such as the Caret package), which has led to their increasingly broad use among users of remote sensing images.
Going beyond detecting vegetation
Remote sensing images allows not only the simple detection of vegetation, but also estimating the properties and qualitative aspects of vegetation that are relevant in various types of monitoring, such as:
- Height of the vegetation;
- Plant vigor;
- Successional stage;
- Water and nutrient stress;
- Biomass and carbon content; and
- Identification of species.
The analysis of the properties and the detection of the characteristics listed above are performed by applying models that relate them to the signal measured by the satellite and also to Vegetation Indexes (VI) that can be calculated from the images.
One of the best known vegetation indexes is NDVI (Normalized Difference Vegetation Index), which is broadly applied to various studies of vegetation by remote sensing. To do so, it is always necessary to collect data in the field that is used to calibrate and train the models selected for application.
Application developed for purposes of environmental licensing for the construction of a real estate development and to manage sustainable use of the site.
The CERTI Staff is prepared to develop solutions in vegetation monitoring using state of the art methods for acquisition and processing of satellite data.
If you would like to learn more about the projects undertaken, contact us: negocios@certi.org.br